A randomized control trial (RCT) is a type of study design that involves randomly assigning participants to either an experimental group or a control group to measure the effectiveness of an intervention or treatment.
Randomized Controlled Trials (RCTs) are considered the “gold standard” in medical and health research due to their rigorous design.
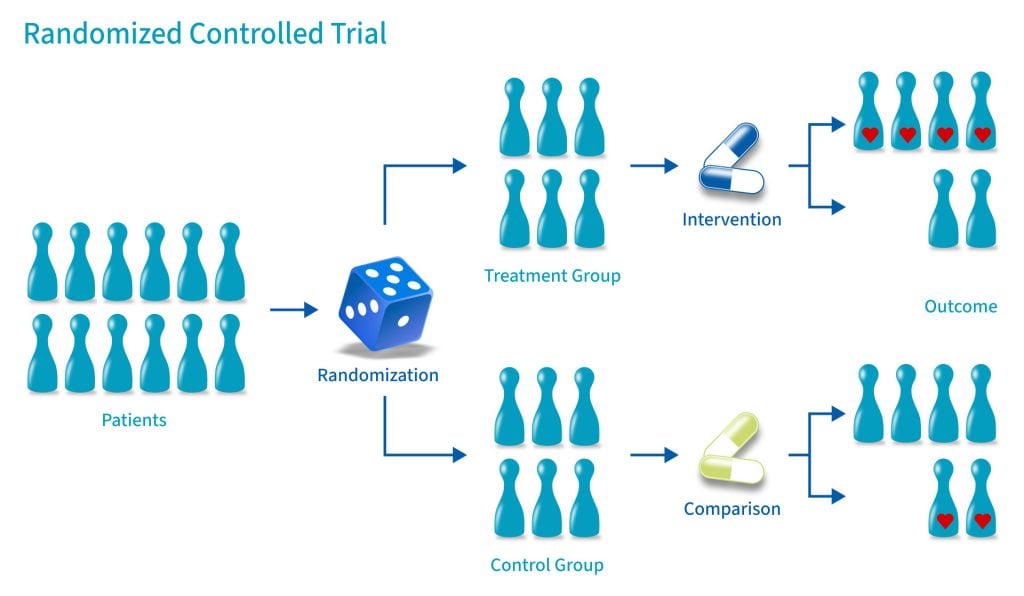
Control Group
A control group consists of participants who do not receive any treatment or intervention but a placebo or reference treatment. The control participants serve as a comparison group.
The control group is matched as closely as possible to the experimental group, including age, gender, social class, ethnicity, etc.
Because the participants are randomly assigned, the characteristics between the two groups should be balanced, enabling researchers to attribute any differences in outcome to the study intervention.
Since researchers can be confident that any differences between the control and treatment groups are due solely to the effects of the treatments, scientists view RCTs as the gold standard for clinical trials.
Random Allocation
Random allocation and random assignment are terms used interchangeably in the context of a randomized controlled trial (RCT).
Both refer to assigning participants to different groups in a study (such as a treatment group or a control group) in a way that is completely determined by chance.
The process of random assignment controls for confounding variables, ensuring differences between groups are due to chance alone.
Without randomization, researchers might consciously or subconsciously assign patients to a particular group for various reasons.
Several methods can be used for randomization in a Randomized Control Trial (RCT). Here are a few examples:
- Simple Randomization: This is the simplest method, like flipping a coin. Each participant has an equal chance of being assigned to any group. This can be achieved using random number tables, computerized random number generators, or drawing lots or envelopes.
- Block Randomization: In this method, participants are randomized within blocks, ensuring that each block has an equal number of participants in each group. This helps to balance the number of participants in each group at any given time during the study.
- Stratified Randomization: This method is used when researchers want to ensure that certain subgroups of participants are equally represented in each group. Participants are divided into strata, or subgroups, based on characteristics like age or disease severity, and then randomized within these strata.
- Cluster Randomization: In this method, groups of participants (like families or entire communities), rather than individuals, are randomized.
- Adaptive Randomization: In this method, the probability of being assigned to each group changes based on the participants already assigned to each group. For example, if more participants have been assigned to the control group, new participants will have a higher probability of being assigned to the experimental group.
Computer software can generate random numbers or sequences that can be used to assign participants to groups in a simple randomization process.
For more complex methods like block, stratified, or adaptive randomization, computer algorithms can be used to consider the additional parameters and ensure that participants are assigned to groups appropriately.
Using a computerized system can also help to maintain the integrity of the randomization process by preventing researchers from knowing in advance which group a participant will be assigned to (a principle known as allocation concealment). This can help to prevent selection bias and ensure the validity of the study results.
Allocation Concealment
Allocation concealment is a technique to ensure the random allocation process is truly random and unbiased.
RCTs use allocation concealment to decide which patients get the real medicine and which get a placebo (a fake medicine)
It involves keeping the sequence of group assignments (i.e., who gets assigned to the treatment group and who gets assigned to the control group next) hidden from the researchers before a participant has enrolled in the study.
This helps to prevent the researchers from consciously or unconsciously selecting certain participants for one group or the other based on their knowledge of which group is next in the sequence.
Allocation concealment ensures that the investigator does not know in advance which treatment the next person will get, thus maintaining the integrity of the randomization process.
Blinding (Masking)
Binding, or masking, refers to withholding information regarding the group assignments (who is in the treatment group and who is in the control group) from the participants, the researchers, or both during the study.
A blinded study prevents the participants from knowing about their treatment to avoid bias in the research. Any information that can influence the subjects is withheld until the completion of the research.
Blinding can be imposed on any participant in an experiment, including researchers, data collectors, evaluators, technicians, and data analysts.
Good blinding can eliminate experimental biases arising from the subjects’ expectations, observer bias, confirmation bias, researcher bias, observer’s effect on the participants, and other biases that may occur in a research test.
In a double-blind study, neither the participants nor the researchers know who is receiving the drug or the placebo. When a participant is enrolled, they are randomly assigned to one of the two groups. The medication they receive looks identical whether it’s the drug or the placebo.
Figure 1 . Evidence-based medicine pyramid. The levels of evidence are appropriately represented by a pyramid as each level, from bottom to top, reflects the quality of research designs (increasing) and quantity (decreasing) of each study design in the body of published literature. For example, randomized control trials are higher quality and more labor intensive to conduct, so there is a lower quantity published.
Resesearch Designs
The choice of design should be guided by the research question, the nature of the treatments or interventions being studied, practical considerations (like sample size and resources), and ethical considerations (such as ensuring all participants have access to potentially beneficial treatments).
The goal is to select a design that provides the most valid and reliable answers to your research questions while minimizing potential biases and confounds.
1. Between-participants randomized designs
Between-participant design involves randomly assigning participants to different treatment conditions. In its simplest form, it has two groups: an experimental group receiving the treatment and a control group.
With more than two levels, multiple treatment conditions are compared. The key feature is that each participant experiences only one condition.
This design allows for clear comparison between groups without worrying about order effects or carryover effects.
It’s particularly useful for treatments that have lasting impacts or when experiencing one condition might influence how participants respond to subsequent conditions.
Example
A study testing a new antidepressant medication might randomly assign 100 participants to either receive the new drug or a placebo.
The researchers would then compare depression scores between the two groups after a specified treatment period to determine if the new medication is more effective than the placebo.
Use this design when:
- You want to compare the effects of different treatments or interventions
- Carryover effects are likely (e.g., learning effects or lasting physiological changes)
- The treatment effect is expected to be permanent
- You have a large enough sample size to ensure groups are equivalent through randomization
2. Factorial designs
Factorial designs investigate the effects of two or more independent variables simultaneously. They allow researchers to study both main effects of each variable and interaction effects between variables.
These can be between-participants (different groups for each combination of conditions), within-participants (all participants experience all conditions), or mixed (combining both approaches).
Factorial designs allow researchers to examine how different factors combine to influence outcomes, providing a more comprehensive understanding of complex phenomena.
They’re more efficient than running separate studies for each variable and can reveal important interactions that might be missed in simpler designs.
Example
A study examining the effects of both exercise intensity (high vs. low) and diet type (high-protein vs. high-carb) on weight loss might use a 2×2 factorial design.
Participants would be randomly assigned to one of four groups: high-intensity exercise with high-protein diet, high-intensity exercise with high-carb diet, low-intensity exercise with high-protein diet, or low-intensity exercise with high-carb diet.
Use this design when:
- You want to study the effects of multiple independent variables simultaneously
- You’re interested in potential interactions between variables
- You want to increase the efficiency of your study by testing multiple hypotheses at once
3. Cluster randomized designs
In cluster randomized trials, groups or “clusters” of participants are randomized to treatment conditions, rather than individuals.
This is often used when individual randomization is impractical or when the intervention is naturally applied at a group level.
It’s particularly useful in educational or community-based research where individual randomization might be disruptive or lead to treatment diffusion.
Example:
A study testing a new teaching method might randomize entire classrooms to either use the new method or continue with the standard curriculum.
The researchers would then compare student outcomes between the classrooms using the different methods, rather than randomizing individual students.
Use this design when:
- You have a smaller sample size available
- Individual differences are likely to be large
- The effects of the treatment are temporary
- You can effectively control for order and carryover effects
4. Within-participants (repeated measures) designs
In these designs, each participant experiences all treatment conditions, serving as their own control.
Within-participants designs are more statistically powerful as they control for individual differences. They require fewer participants, making them more efficient.
However, they’re only appropriate when the treatment effects are temporary and when you can effectively counterbalance to control for order effects.
Example
A study on the effects of caffeine on cognitive performance might have participants complete cognitive tests on three separate occasions: after consuming no caffeine, a low dose of caffeine, and a high dose of caffeine.
The order of these conditions would be counterbalanced across participants to control for order effects.
Use this design when:
- You have a smaller sample size available
- Individual differences are likely to be large
- The effects of the treatment are temporary
- You can effectively control for order and carryover effects
5. Crossover designs
Crossover designs are a specific type of within-participants design where participants receive different treatments in different time periods.
This allows each participant to serve as their own control and can be more efficient than between-participants designs.
Crossover designs combine the benefits of within-participants designs (increased power, control for individual differences) with the ability to compare different treatments.
They’re particularly useful in clinical trials where you want each participant to experience all treatments, but need to ensure that the effects of one treatment don’t carry over to the next.
Example:
A study comparing two different pain medications might have participants use one medication for a month, then switch to the other medication for another month after a washout period.
Pain levels would be measured during both treatment periods, allowing for within-participant comparisons of the two medications’ effectiveness.
Use this design when:
- You want to compare the effects of different treatments within the same individuals
- The treatments have temporary effects with a known washout period
- You want to increase statistical power while using a smaller sample size
- You want to control for individual differences in response to treatment
Advantages
Prevents bias
In randomized control trials, participants must be randomly assigned to either the intervention group or the control group, such that each individual has an equal chance of being placed in either group.
This is meant to prevent selection bias and allocation bias and achieve control over any confounding variables to provide an accurate comparison of the treatment being studied.
Because the distribution of characteristics of patients that could influence the outcome is randomly assigned between groups, any differences in outcome can be explained only by the treatment.
High statistical power
Because the participants are randomized and the characteristics between the two groups are balanced, researchers can assume that if there are significant differences in the primary outcome between the two groups, the differences are likely to be due to the intervention.
This warrants researchers to be confident that randomized control trials will have high statistical power compared to other types of study designs.
Blinding
Since the focus of conducting a randomized control trial is eliminating bias, blinded RCTs can help minimize any unconscious information bias.
In a blinded RCT, the participants do not know which group they are assigned to or which intervention is received. This blinding procedure should also apply to researchers, health care professionals, assessors, and investigators when possible.
“Single-blind” refers to an RCT where participants do not know the details of the treatment, but the researchers do.
“Double-blind” refers to an RCT where both participants and data collectors are masked of the assigned treatment.
Limitations
Costly and Timely
Some interventions require years or even decades to evaluate, rendering them expensive and time-consuming.
It might take an extended period of time before researchers can identify a drug’s effects or discover significant results.
Requires large sample size
There must be enough participants in each group of a randomized control trial so researchers can detect any true differences or effects in outcomes between the groups.
Researchers cannot detect clinically important results if the sample size is too small.
Change in population over time
Because randomized control trials are longitudinal in nature, it is almost inevitable that some participants will not complete the study, whether due to death, migration, non-compliance, or loss of interest in the study.
This tendency is known as selective attrition and can threaten the statistical power of an experiment.
Ethics
Randomized control trials are not always practical or ethical, and such limitations can prevent researchers from conducting their studies.
For example, a treatment could be too invasive, or administering a placebo instead of an actual drug during a trial for treating a serious illness could deny a participant’s normal course of treatment. Without ethical approval, a randomized control trial cannot proceed.
Fictitious Example
An example of an RCT would be a clinical trial comparing a drug’s effect or a new treatment on a select population.
The researchers would randomly assign participants to either the experimental group or the control group and compare the differences in outcomes between those who receive the drug or treatment and those who do not.
Real-life Examples
- Preventing illicit drug use in adolescents: Long-term follow-up data from a randomized control trial of a school population (Botvin et al., 2000).
- A prospective randomized control trial comparing medical and surgical treatment for early pregnancy failure (Demetroulis et al., 2001).
- A randomized control trial to evaluate a paging system for people with traumatic brain injury (Wilson et al., 2009).
- Prehabilitation versus Rehabilitation: A Randomized Control Trial in Patients Undergoing Colorectal Resection for Cancer (Gillis et al., 2014).
- A Randomized Control Trial of Right-Heart Catheterization in Critically Ill Patients (Guyatt, 1991).
- Preventing illicit drug use in adolescents: Long-term follow-up data from a randomized control trial of a school population (Botvin et al., 2000).
- Berry, R. B., Kryger, M. H., & Massie, C. A. (2011). A novel nasal excitatory positive airway pressure
(EPAP) device for the treatment of obstructive sleep apnea: A randomized controlled trial. Sleep, 34,
479–485. - Gloy, V. L., Briel, M., Bhatt, D. L., Kashyap, S. R., Schauer, P. R., Mingrone, G., . . . Nordmann, A. J. (2013,
October 22). Bariatric surgery versus non-surgical treatment for obesity: A systematic review and
meta-analysis of randomized controlled trials. BMJ, 347. - Streeton, C., & Whelan, G. (2001). Naltrexone, a relapse prevention maintenance treatment of alcohol
dependence: A meta-analysis of randomized controlled trials. Alcohol and Alcoholism, 36 (6), 544–552.
How Should an RCT be Reported?
Reporting of a Randomized Controlled Trial (RCT) should be done in a clear, transparent, and comprehensive manner to allow readers to understand the design, conduct, analysis, and interpretation of the trial.
The Consolidated Standards of Reporting Trials (CONSORT) statement is a widely accepted guideline for reporting RCTs.
Further Information
- Cocks, K., & Torgerson, D. J. (2013). Sample size calculations for pilot randomized trials: a confidence interval approach. Journal of clinical epidemiology, 66(2), 197-201.
- Kendall, J. (2003). Designing a research project: randomised controlled trials and their principles. Emergency medicine journal: EMJ, 20(2), 164.
References
Akobeng, A.K., Understanding randomized controlled trials. Archives of Disease in Childhood, 2005; 90: 840-844.
Bell, C. C., Gibbons, R., & McKay, M. M. (2008). Building protective factors to offset sexually risky behaviors among black youths: a randomized control trial. Journal of the National Medical Association, 100 (8), 936-944.
Bhide, A., Shah, P. S., & Acharya, G. (2018). A simplified guide to randomized controlled trials. Acta obstetricia et gynecologica Scandinavica, 97 (4), 380-387.
Botvin, G. J., Griffin, K. W., Diaz, T., Scheier, L. M., Williams, C., & Epstein, J. A. (2000). Preventing illicit drug use in adolescents: Long-term follow-up data from a randomized control trial of a school population. Addictive Behaviors, 25 (5), 769-774.
Demetroulis, C., Saridogan, E., Kunde, D., & Naftalin, A. A. (2001). A prospective randomized control trial comparing medical and surgical treatment for early pregnancy failure. Human Reproduction, 16 (2), 365-369.
Gillis, C., Li, C., Lee, L., Awasthi, R., Augustin, B., Gamsa, A., … & Carli, F. (2014). Prehabilitation versus rehabilitation: a randomized control trial in patients undergoing colorectal resection for cancer. Anesthesiology, 121 (5), 937-947.
Globas, C., Becker, C., Cerny, J., Lam, J. M., Lindemann, U., Forrester, L. W., … & Luft, A. R. (2012). Chronic stroke survivors benefit from high-intensity aerobic treadmill exercise: a randomized control trial.
Neurorehabilitation and Neural Repair, 26
(1), 85-95.
Guyatt, G. (1991). A randomized control trial of right-heart catheterization in critically ill patients. Journal of Intensive Care Medicine, 6 (2), 91-95.
MediLexicon International. (n.d.). Randomized controlled trials: Overview, benefits, and limitations. Medical News Today. Retrieved from https://www.medicalnewstoday.com/articles/280574#what-is-a-randomized-controlled-trial
Wilson, B. A., Emslie, H., Quirk, K., Evans, J., & Watson, P. (2005). A randomized control trial to evaluate a paging system for people with traumatic brain injury. Brain Injury, 19 (11), 891-894.